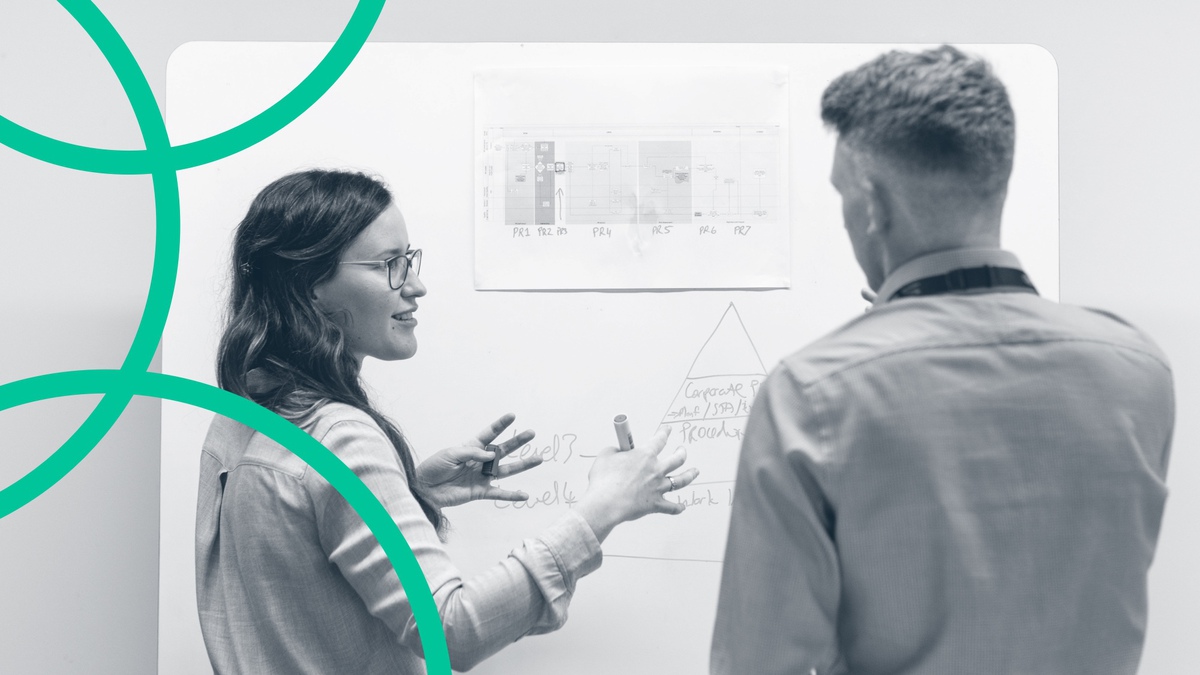
Towards solving the replication crisis
Earlier this year, we hosted a webinar in collaboration with the National Organization for Research Development Professionals (NORDP). In“The Role of RD in Research Integrity” we discussed current issues surrounding rigor, transparency, and the replication crisis in scholarly publications and the role of research development professionals in supporting its evolution. In this blog post, we’ll share some of the key points raised during the webinar and what F1000 is doing to support research institutions in their efforts to enhance rigor and replicability.
Is there a replication crisis?
According to an online survey of academic researchers hosted by Nature in 2016, a staggering 90% of those surveyed believed there is a reproducibility crisis. Plus, only 7% were unsure and 52% labeled the crisis as ‘significant.’ Perhaps it’s not surprising then, that the vast majority of the survey’s respondents indicated that they had personally failed to reproduce another scientist’s results. Plus, as many as 65% admitted to having failed to reproduce their own research results.
So, what contributes to the replication crisis? A number of barriers are detrimental to replicability, including:
- Selective reporting – the idea that researchers are only publishing their most novel or positive findings
- Lack of methods – without a clear articulation of a study’s methods, it can be nearly impossible to replicate results
- Poor experimental design
- Lack of raw data availability from the original lab
In an editorial published in Molecular Brain, Tsuyoshi Miyakawa (2020) argues that the replication crisis results from the practice of publishing research without the corresponding raw data. Miyakawa suggests:
“The idea of open science/open data is needed [to solve the replication crisis]…and I propose that a custom should be commonly accepted, that sharing raw data publicly is a necessary condition for a study to be considered as scientifically sound, unless the authors have acceptable reasons not to do so (e.g., data contains confidential personal information).”
Tsuyoshi Miyakawa
Open research and FAIR data principles
FAIR data principles and open research are two broad mechanisms designed to improve research rigor and replicability. Open research refers to a set of principles and practices that prioritize openness, including transparency across all stages of the research lifecycle. This includes experiments, data, and other research outputs. Furthermore, open research supports research integrity at its highest level, providing sufficient information to ensure published research withstands scientific rigor, while also supporting researchers with enhanced collaboration opportunities. As a result, this strengthens the reach and academic record for their research and creates additional attribution opportunities by exposing more of their research to interrogation.
FAIR data principles, introduced in 2016 and widely adopted worldwide, mean that the data that underlies scholarly research must be:
- Findable: Metadata and data used in the research should be easy to find – for both humans and computers. This can be achieved by assigning a persistent identifier, such as a DOI, to the data and providing machine-readable metadata.
- Accessible: Data should be easy to access once it has been found. For sensitive research data, this may mean implementing an authentication and authorization procedure.
- Interoperable: Ensure your data can be easily integrated with other data. Research data should use an accessible, shared language for knowledge representation, and use vocabularies that follow FAIR principles as well.
- Reusable: To enable reuse, research data and metadata should have rich, meaningful descriptions, aimed at helping others with replication. Research data should meet any discipline-specific standards, and should be released under a clear, accessible license.
As a result, FAIR data coupled with the attributes of open research offer meaningful solutions to aid reproducibility within the mounting replication crisis. In the webinar, Joseph and Dr. Eck explained why FAIR data sharing is integral to enhancing rigor and replicability.
F1000: Open research solutions
Publishing all underlying research data alongside traditional research articles, including Data Sets, Genome Notes, Posters, and other research outputs improves the academic record.
Unlike traditional publishing, F1000’s open publishing model introduces more research outputs to the rigors of scientific inquiry. Researchers can tell the full story of their research through a variety of article types, including Brief Reports, Data Notes, Case Studies, and more. Such options enable all parts of a research project to be discovered, used, reproduced, and cited. Furthermore, many of the article types published with F1000 are peer reviewed, contributing to academic rigor.
Interested in hearing more about how F1000 partners with institutions to support enhanced rigor and reproducibility? Watch ‘The Role of RD in Research Integrity’ webinar recording on-demand now.