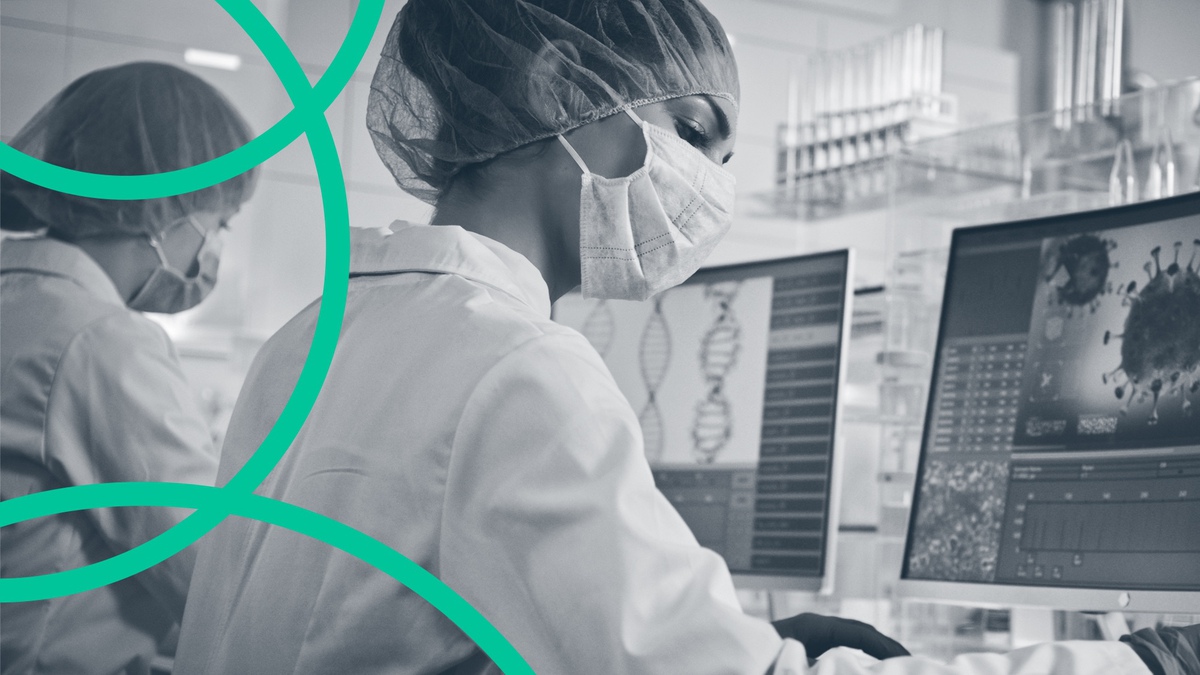
Data management supporting the research communications ecosystem
At F1000 we foster a culture of innovation to accelerate the reach of knowledge and put it in the hands of those who will shape the future. We are committed to ensuring that the partners we work with are empowered with the right knowledge and skills in order to achieve their open research ambitions. To help further this mission, Dr. Rebecca Grant, Head of Data and Software Publishing at F1000, and Matt Cannon, Head of Open Research at Taylor & Francis, hosted a workshop at the AAAS Annual Meeting. As research data experts, they explored the topic of data management within the increasingly open and collaborative research communications ecosystem.
In this blog, Rebecca discusses why it is so important for researchers to share their data, how to overcome the challenges of sharing data and how data sharing can be easily embedded into the research workflow.
“Sharing research data is much easier when researchers prepare for it in advance. Thinking about elements of data management like data storage and formats at the start of a project can save lots of time when it comes to publishing the research article.”
Dr. Rebecca Grant, Head of Data and Software Publishing at F1000
Encouraging a culture of data sharing
At F1000, we want academic research to have a real-world impact. Increasingly stakeholders are recognizing the central role of accessible research data to achieve this ambition: by making it easier to question, share, replicate, validate, confirm, or build on the evidence which supports a piece of research.
We believe that for a scholarly communications ecosystem supported by research data to develop and thrive, data shouldn’t be left on an old hard drive or filed away in a locked drawer. Ultimately, we believe that research data should be as open as possible, (and as closed as necessary) and align with the FAIR principles (Findable, Accessible, Interoperable, and Reproducible).
Data management is essential to the research lifecycle
Research data can be the input to or output of the research process. When publishers ask researchers to share their research data, we are usually most interested in the portion of the data that supports the conclusion of the published research article. Depending on the discipline and research methods, the dataset can take many forms: it could be a gene sequence, a spreadsheet, interview notes, transcripts, images, or even audio-visual materials.
Regardless of the format, data sharing is key to producing transparent, reproducible research. Having access to research data helps peer reviewers and readers to understand the research process and how the research findings were established. Additionally, sharing reusable, openly licensed data allows others to build on this work and prevents duplication of effort – if a dataset has been shared, it doesn’t need to be recreated. That’s why other stakeholders, like research funders, encourage researchers to share their data openly.
Ideally, data should be shared in a way that ensures its accessibility in the long-term; that allows the creator to assign an open license; that helps others to find the data; and that provides researchers with a persistent identifier (like a DOI, Digital Object Identifier), therefore, the data can be cited. Data repositories are the best way to achieve all of these aims but we appreciate that getting the dataset into a repository can be more difficult if it wasn’t planned for in advance. This is why research data management is so beneficial.
Research data management is a series of processes that researchers undertake to ensure that they come to the end of the research project with well-annotated data which has been stored securely. It should be planned for and undertaken actively while the research is in progress. Along the way, researchers will consider aspects like what file formats they’ll create, how they’ll store the data in the short and long-term, whether they’ll be able to apply for a particular license and how they’ll capture any contextual information about how the data was generated.
The process will look different depending on the discipline, the team or lab the researchers are working with, and the type of research they’re doing.
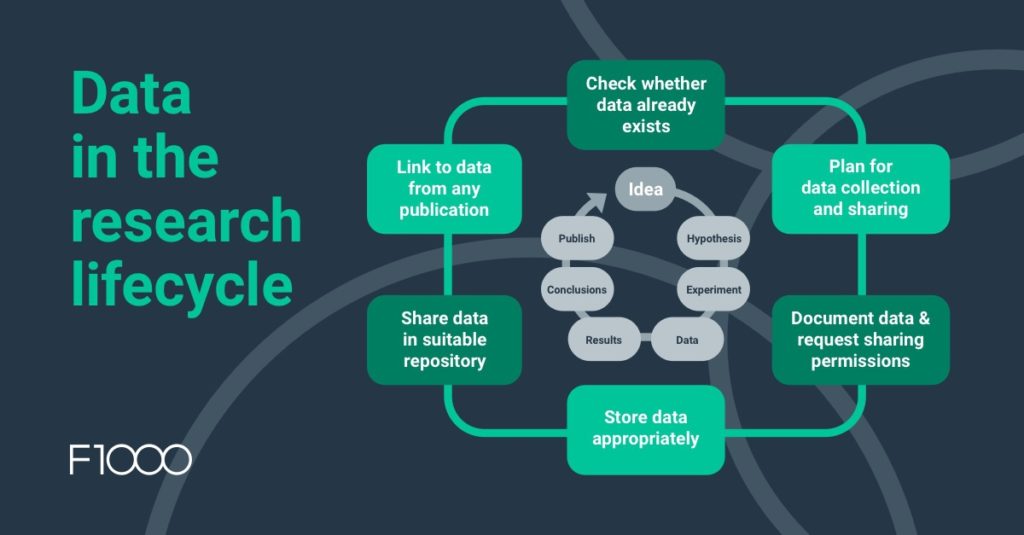
Data management across every stage of the research lifecycle
The research lifecycle can look slightly different for different researchers, but generally, it refers to a series of steps where the researcher identifies an idea or research question; defines their hypothesis; conducts their experiment; generates and analyses their data; and establishes their research findings or results. At the end of the cycle, most researchers will choose to publish an output, such as a journal article, and the cycle begins again with a new piece of research which may build on their findings. This is important in a research data sharing context as there are additional decisions which should be made at different points to ensure that the data generated can be shared appropriately. For example, a researcher who works with human research participants will need to get informed consent from the participants to allow the data to be shared at the end of the project. This should be done early on when the participants are being recruited – once they’re at the end of the project and about to publish, it’s too late.
Data management planning can also help you to decide which parts of the data to share. Ideally, most researchers would share a large proportion of the data they generate; even if it is ‘null’ data (e.g., that didn’t support their initial hypothesis). Researchers never know what someone might reuse the data for! Most funding agency policies on data sharing will prompt researchers to share most or all their data.
Publishers are more concerned with the portion of the data that underpins the results of the study the researchers are publishing, however – that’s what we expect from authors at F1000. Our F1000 Open data policy requires that the data shared by authors is ‘open data’. ‘Open data’ refers to data which is both openly accessible (in a data repository) and openly licensed for reuse.
Overcoming the challenges in data sharing
The challenges encountered by researchers will depend on the type of data that is generated in the research. Researchers who base their study on sources which are in copyright (for example in the humanities), may not have permission to re-share their data with an open license applied. Anyone working with human research participants will have both ethical and legal elements to consider, ensuring the data can be shared safely.
Therefore, it’s important to begin thinking about data sharing at the start of the research process and to embed research data management across every stage of the research lifecycle. If researchers have established the challenges or limitations early on, they can consider the best way to mitigate them before it comes to the end of the project and find that they are unable to comply with any data-sharing policies that might apply to them.
One aspect of data sharing which some authors may struggle with is identifying suitable research data repositories. There are thousands of data repositories available which span all disciplines. Both www.re3data.org and www.fairsharing.org provide searchable indexes of data repositories which can be browsed or searched.
For F1000 authors, we recommend using one of our approved repositories, which have been assessed to ensure that they provide key functionality such as long-term storage and the provision of persistent identifiers. As an advocate for open data, we have additional guidance on all aspects of research data management and sharing available on our website.
Find out more about our open research solutions here.
About Rebecca Grant
Dr. Rebecca Grant, Head of Data and Software Publishing at F1000, is a qualified data trainer certified by the Open Data Institute. Her doctoral thesis explored the connections between archival theory and research data management practice.